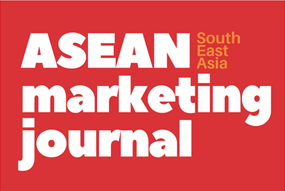
Abstract
Manuscript Type: Research Article
Research Aims: This research is expected to contribute to scientific insights on machine learning predictions, how to understand the contribution of variables and their interpretation in the overall context of customer churn in telecommunications companies.
Design/Methodology/Approach: Data was collected from one Indonesian telecommunication company within a total of 50,000 sample data points. The data was analyzed using a machine learning algorithm to process, predict, and interpret the result based on the research scenario.
Research Findings: These findings revealed that the SHAP framework significantly impacts the churn problem, allowing the marketing team to implement the right strategy based on customer personalization.
Theoretical Contribution/Originality: This research enriches customer churn research in the telecommunications industry by introducing a combined method of the LightGBM model and SHAP framework, providing a thorough analysis of variable contributions to the customer churn model used, and addressing the lack of research that focuses on variable contributions.
Practitioner/Policy Implication: This research provides an overview of the utilization of customer variables that can later be studied deeply by data or marketing teams to produce initiative projects based on data and machine learning models
Research Limitation/Implication: Future studies could combine the feature selection method to filter the model’s features and remove redundant ones, thereby analysing the contribution of variables that truly impact customer churn.
Keywords: telecommunication; customer churn; machine learning; shap framework; lightgbm
Bahasa Abstract
Jenis Naskah: Artikel Penelitian
Tujuan Penelitian: Penelitian ini diharapkan dapat memberikan kontribusi pada wawasan ilmiah tentang prediksi machine learning, bagaimana memahami kontribusi variabel dan interpretasinya dalam konteks keseluruhan churn pelanggan di perusahaan telekomunikasi.
Desain/Metodologi/Pendekatan: Data dikumpulkan dari satu perusahaan telekomunikasi di Indonesia dengan total 50.000 titik data sampel. Data dianalisis menggunakan algoritma machine learning untuk memproses, memprediksi, dan menginterpretasikan hasil berdasarkan skenario penelitian.
Temuan Penelitian: Temuan ini mengungkapkan bahwa kerangka kerja SHAP secara signifikan berdampak pada masalah churn, memungkinkan tim pemasaran untuk menerapkan strategi yang tepat berdasarkan personalisasi pelanggan.
Kontribusi Teoritis/Originalitas: Penelitian ini memperkaya penelitian customer churn di industri telekomunikasi dengan memperkenalkan metode gabungan dari model LightGBM dan kerangka kerja SHAP, memberikan analisis menyeluruh mengenai kontribusi variabel terhadap model customer churn yang digunakan, dan mengatasi kurangnya penelitian yang berfokus pada kontribusi variabel.
Implikasi Praktisi/Kebijakan: Penelitian ini memberikan gambaran mengenai pemanfaatan variabel pelanggan yang nantinya dapat dipelajari secara mendalam oleh tim data atau pemasaran untuk menghasilkan proyek inisiatif yang berbasis data dan model machine learning
Keterbatasan Penelitian/Implikasi: Penelitian selanjutnya dapat menggabungkan metode seleksi fitur untuk menyaring fitur-fitur model dan menghilangkan fitur yang berlebihan, sehingga dapat menganalisis kontribusi variabel yang benar-benar berdampak pada customer churn.
Kata kunci: telekomunikasi; perpindahan pelanggan; pembelajaran mesin; kerangka kerja shap; lightgbm
References
Abdulsalam, S. O., Arowolo, M. O., & Saheed, Y. K. (2022). Customer churn prediction in the telecommunication industry using classification and regression trees and artificial neural network algorithms. International Journal of Electrical and Electronic Engineering & Telecommunications, 10(2). https://doi.org/10.52549/ijeei.v10i2.2985
Al Majzoub, H., Elgedawy, I., Akaydın, Ö., & Köse Ulukök, M. (2020). HCAB-SMOTE: A hybrid clustered affinitive borderline SMOTE approach for imbalanced data binary classification. Arabian Journal for Science and Engineering, 45(4), 3205–3222. https://doi.org/10.1007/s13369-019-04336-1
Alboukaey, N., Joukhadar, A., & Ghneim, N. (2020). Dynamic behavior based churn prediction in mobile telecom. Expert Systems with Applications, 162, 113779. https://doi.org/10.1016/j.eswa.2020.113779
Alimi, O. A., Ouahada, K., Abu-Mahfouz, A. M., Rimer, S., & Alimi, K. O. A. (2021). A review of research works on supervised learning algorithms for SCADA intrusion detection and classification. Sustainability, 13(17), 1–19. https://doi.org/10.3390/su13179597
Arai, K., Fujikawa, I., Nakagawa, Y., Momozaki, R., & Ogawa, S. (2023). Churn customer estimation method based on LightGBM for improving sales. International Journal of Electrical and Electronic Engineering & Telecommunications, 14(2), 119–126.
Banu, J. F., Neelakandan, S., Geetha, B. T., Selvalakshmi, V., Umadevi, A., & Martinson, E. O. (2022). Artificial intelligence-based customer churn prediction model for business markets. Computational Intelligence and Neuroscience, 1-14.
Calzada-Infante, L., Óskarsdóttir, M., & Baesens, B. (2020). Evaluation of customer behavior with temporal centrality metrics for churn prediction of prepaid contracts. Expert Systems with Applications, 160, 113553. https://doi.org/10.1016/j.eswa.2020.113553
Jafari, M. J., Tarokh, M. J., & Soleimani, P. (2023). An interpretable machine learning framework for customer churn prediction: A case study in the telecommunications industry. Journal of Industrial Engineering and Management Studies, 10(1), 141–157. https://doi.org/10.22116/jiems.2023.365114.1504
Ke, G., Meng, Q., Finley, T., Wang, T., Chen, W., Ma, W., Ye, Q., & Liu, T. Y. (2017). LightGBM: A highly efficient gradient boosting decision tree. Advances in Neural Information Processing Systems, 2017-Decem(NIPS), 3147–3155.
Khraisat, A., Gondal, I., Vamplew, P., & Kamruzzaman, J. (2019). Survey of intrusion detection systems: techniques, datasets and challenges. Cybersecurity, 2(1). https://doi.org/10.1186/s42400-019-0038-7
Kovács, B., Tinya, F., Németh, C., & Ódor, P. (2020). Unfolding the effects of different forestry treatments on microclimate in oak forests: Results of a 4-year experiment. Ecological Applications, 30(2), 321–357. https://doi.org/10.1002/eap.2043
Lalwani, P., Mishra, M. K., Chadha, J. S., & Sethi, P. (2022). Customer churn prediction system: A machine learning approach. Computing, 104(2), 271–294. https://doi.org/10.1007/s00607-021-00908-y
Liang, W., Luo, S., Zhao, G., & Wu, H. (2020). Predicting hard rock pillar stability using GBDT, XGBoost, and LightGBM algorithms. Mathematics, 8(5), 1–17. https://doi.org/10.3390/math8050765
Lundberg, S. M., & Lee, S. I. (2017). A unified approach to interpreting model predictions. Advances in Neural Information Processing Systems, 2017-Decem(3), 4766–4775.
Moustakidis, S., Plakias, S., Kokkotis, C., Tsatalas, T., & Tsaopoulos, D. (2023). Predicting football team performance with explainable AI: Leveraging SHAP to identify key team-level performance metrics. Future Internet, 15(5). https://doi.org/10.3390/fi15050174
Peng, K., & Peng, Y. (2022). Research on telecom customer churn prediction based on GA-XGBoost and SHAP. Journal of Computer and Communications, 10(1), 107–120. https://doi.org/10.4236/jcc.2022.1011008
Rufo, D. D., Debelee, T. G., Ibenthal, A., & Negera, W. G. (2021). Diagnosis of diabetes mellitus using gradient boosting machine (LightGBM). Diagnostics, 11(9), 1–14. https://doi.org/10.3390/diagnostics11091714
Tang, Q., Xia, G., Zhang, X., & Long, F. (2020). A customer churn prediction model based on XGBoost and MLP. Proceedings - 2020 International Conference on Computer Engineering and Application (ICCEA 2020), 608–612. https://doi.org/10.1109/ICCEA50009.2020.00133
Vo, N. N. Y., Liu, S., Li, X., & Xu, G. (2021). Leveraging unstructured call log data for customer churn prediction. Knowledge-Based Systems, 212, 106586. https://doi.org/10.1016/j.knosys.2020.106586
Zeng, Q., Chang, M., Tong, Q., & Su, J. (2023). Retracted: A prediction model of customer churn considering customer value: An empirical research of telecom. Discrete Dynamics in Nature and Society, vol. 2021.
Zhang, T., Moro, S., & Ramos, R. F. (2022). A data-driven approach to improve customer churn prediction based on telecom customer segmentation. Future Internet, 14(3), 1–19. https://doi.org/10.3390/fi14030094
Recommended Citation
Sinaga, Ruben and Widianto, Sunu
(2023)
"Understanding Telecommunication Customer Churn: Insights from LightGBM Predictive Modelling and SHAP Feature Interpretation,"
ASEAN Marketing Journal: Vol. 15:
No.
1, Article 4.
DOI: 10.7454/amj.v15i1.1229
Available at:
https://scholarhub.ui.ac.id/amj/vol15/iss1/4